Overview
This document provides an overview of the surface temperature products (T2m) of ASRv2. ASRv2 is a multi-agency, university-led regional reanalysis product that covers the Arctic. It is produced using high-resolution versions of the Polar Weather Forecast Model (PWRF) and the WRF-VAR and High Resolution Land Data Assimilation (HRLDAS) systems that have been optimized for the Arctic. The final version, which has 15 km horizontal resolution and spans 2000-2016 period, is available online through the NCAR's RDA.
Provider's contact information
ASRv2 is produced by Polar Meteorology Group, Byrd Polar & Climate Research Center, the Ohio State University and is available at NCAR CISL RDA.
RDA NCAR user support manager : schuster@ucar.edu
Licensing and citation
Licence: licensed under Creative Commons Attribution 4.0 International Licence (Licence agreement information can be found here)
Dataset citable as:
National Center for Atmospheric Research/University Corporation for Atmospheric Research, and Polar Meterology Group/Byrd Polar and Climate Research Center/The Ohio State University. 2017. Arctic System Reanalysis version 2. Research Data Archive at the National Center for Atmospheric Research, Computational and Information Systems Laboratory. https://doi.org/10.5065/D6X9291B , https://rda.ucar.edu/datasets/ds631.1/
Bromwich, D., L. Bai, K. Hines, S. Wang, Z. Liu, H. Lin, Y. Kuo, and M. Barlage. 2012: Arctic System Reanalysis (ASR) Project. Research Data Archive at the National Center for Atmospheric Research, Computational and Information Systems Laboratory.
Variable name and units:
The 2 m temperature of ASRv2 is the main focus of this annex. This parameter has units of kelvin (K). Temperature measured in kelvin can be converted to degrees Celsius (°C) by subtracting 273.15.
The parameter is noted 2 m temperature and it is available from the ‘’2D surface analysis’’ as well as from the ‘’2D surface forecast’’ datasets. Many other variables are provided in those two groups.
Spatial coverage and resolution:
Geographical Coverage: 15 km x 15 km (at 60 N) oriented 175 W (720 x 720 North Polar Stereographic).
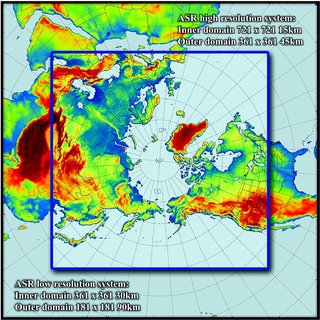
Figure 1. ASR domain (from http://polarmet.osu.edu/ASR/)
Temporal coverage and resolution:
ASRv2 "2 m temperature" is available from 2000 to 2016 as an instantaneous parameter provided at 3 hourly time step and as monthly means.
Methodology
ASRv2 provides a high resolution description of the atmosphere-sea ice-land surface system of the Arctic. It is using the polar version of the Weather Research and Forecasting (WRF) model version 3.6.0. It uses the 3DVAR technique and the High Resolution Land Data Assimilation (HRLDAS) data assimilation systems that have been optimized for the Arctic.
A full description of ASRv2 is presented in the Bulletin of the American Meteorological Society (PDF).
Observations data in ASRv2
The observations data used in ASRv2 (Figure 2) includes synoptic surface observations (black dots), METARs (purple plus signs), ship observations (royal blue dots), buoys (navy-blue dots), radiosondes (purple asterisks), global positioning system refractivity observations (red dots), wind profiler (yellow dots), aviation in-flight weather reports (green dots), QuikSCAT sea surface winds (orange dots), and satellite atmospheric motion vectors (aqua dots).
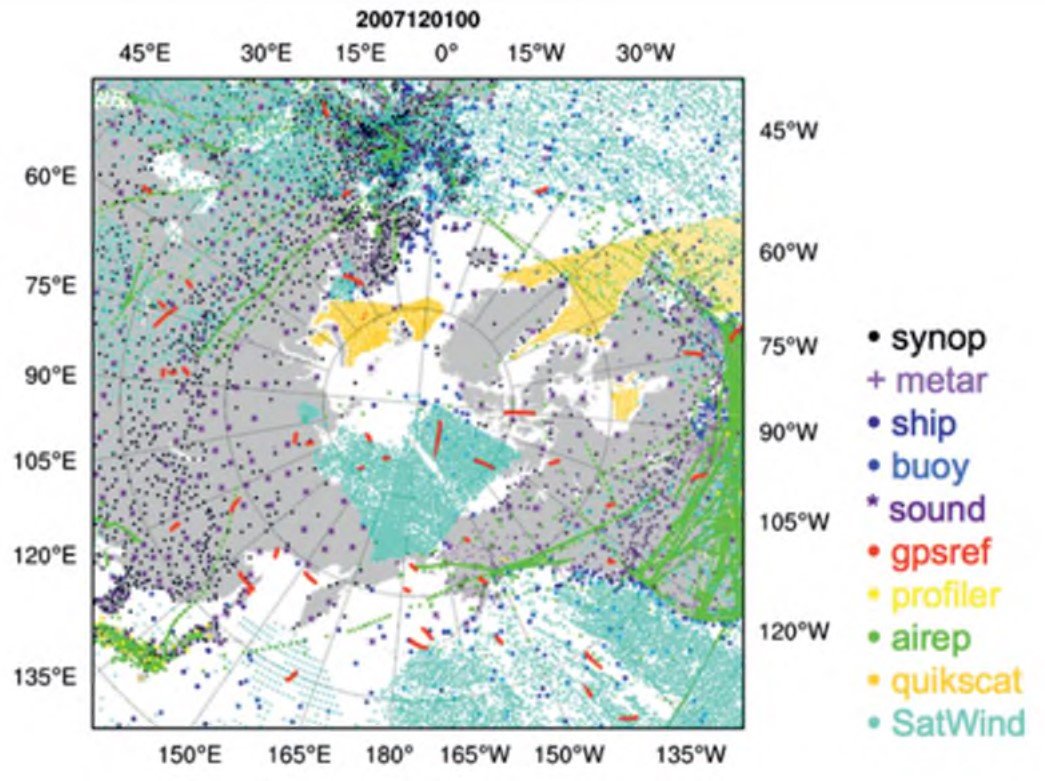
Figure 2. Distribution of observations.
Information about the technical and scientific quality
New features in ASRv2 compared to ASRv1 are higher horizontal resolution, updated model physics including sub-grid scale cloud fraction interaction with radiation, and a dual outer loop routine for more accurate data assimilation.
The following document compares near-surface variables from ASRv1, ASRv2, and ERAI to observations from ∼4500 surface stations provided by the National Centers for Environmental Information (https://www.ncdc.noaa.gov/) for the period December 2006-November 2007: http://polarmet.osu.edu/ASR/asr_v2_table.pdf. For 2 m temperature it is mentioned: ‘’Analysis reveals that ERAI and ASR products have small annual mean biases, with the smallest biases represented by ASRv2. However, ASRv2 is colder than both ASRv1 and ERAI with negative biases from February through October. Decreasing annual mean RMSE values from ERAI to ASRv2 indicate that ASRv2 is an excellent fit to observations and the standard deviation of the unexplained variance is small. This is further supported by increasing skill indicated by higher correlation.’’
Presently there are plans to update ASRv2. The updated version will use the latest version of WRF and WRFDA, a more advanced data assimilation procedure, implement Morrison microphysics with a specified variable aerosol concentration, change to Noah-MP land surface model, incorporate a thermodynamic sea ice model, and increase the horizontal resolution to at least 10 km with ~ 100 vertical levels. This version will be known as ASRv3. Plans are to conduct a reanalysis of the MOSAiC drift period (fall 2019 - fall 2020) and it will be available through NCAR.
Limitations and strengths for application in North Canada
The following notes are general observations provided at https://climatedataguide.ucar.edu/climate-data/arctic-system-reanalysis-asr :
Key Strengths: Excellent reproduction of near-surface and tropospheric variables
Key Limitations: A dry bias is still present during the cooler months in ASRv2.
References to documents describing the methodology or/and the dataset
Bromwich, D., Y.-H. Kuo, M. Serreze, J. Walsh, L.S. Bai, M. Barlage, K. Hines, and A, Slater, 2010: Arctic System Reanalysis: Call for community involvement. EOS Trans. AGU, 91, 13-14. https://doi.org/10.1029/2010EO020001
Online technical description:
https://rda.ucar.edu/datasets/ds631.0/#!docs
Link to download the data and format of data:
Data Access: NCAR/RDA
ASRv2 is available in NetCDF format.
Link to download 3 hourly and monthly data on RDA:
ASR 15 km monthly means of analysis products
ASR 15 km monthly means of forecast products
Publications including dataset evaluation or comparison with other data in Canada
Bromwich, D.H., A.B. Wilson, L. Bai, G.W.K. Moore, and P. Bauer, 2016: A comparison of the regional Arctic System Reanalysis and the global ERA-Interim Reanalysis for the Arctic. Q. J. R. Meteorol. Soc., 142, 644-658. https://doi.org/10.1002/qj.2527
Bromwich, D.H., K.M. Hines, and L.-S. Bai, 2009: Development and testing of Polar WRF: 2. Arctic Ocean. J. Geophys. Res., 114, D08122. https://doi.org/10.1029/2008JD010300
Smirnova, J., and P. Golubkin, 2017: Comparing polar lows in atmospheric reanalyses: Arctic System Reanalysis versus ERA-Interim. Mon. Wea. Rev., 145, 2375-2383, https://doi.org/10.1175/MWR-D-16-0333.1
Avila-Diaz, A., D.H. Bromwich, A.B. Wilson, F. Justino, S.-H. Wang, 2021: Climate extremes across the North American Arctic in modern reanalyses. J. Climate, , 34, 2385–2410, https://doi.org/10.1175/JCLI-D-20-0093.1
Chanona, M., and S. Waterman, 2020: Temporal Variability of Internal Wave-Driven Mixing in Two Distinct Regions of the Arctic Ocean. J. Geophys. Res. Oceans, 125, https://doi.org/10.1029/2020JC016181
Edel, L., C. Claud, C. Genthon, C. Palerme, N. Wood, T. L’Ecuyer, and D. Bromwich, 2020: Arctic snowfall from CloudSat observations and reanalyses. J. Clim., 33, 2093-2109, https://doi.org/10.1175/JCLI-D-19-0105.1
Kaiser-Weiss, A.K., M. Borsche, D. Niermann, F. Kaspar, C. Lussana, F.A. Isotta, E. van den Besselaar, G. van der Schrier, and P. Undén, 2019: Added value of regional reanalyses for climatological applications. Environ. Res. Commun., 1, https://doi.org/10.1088/2515-7620/ab2ec3
Gutjahr, O., and G. Heinemann, 2018: A model-based comparison of extreme winds in the Arctic and around Greenland. Int. J. Clmatol., 38, 5272-5292, https://doi.org/10.1002/joc.5729
Rabatel, M., P. Rampal, A. Carrassi, L. Bertino, and C. K. Jones, 2018: Impact of rheology on probabilistic forecasts of sea ice trajectories: Application for search and rescue operations in the Arctic. Cryosphere, 12, 935-953, https://doi.org/10.5194/tc-12-935-2018
Kohnemann, S.H., G. Heinemann, D.H. Bromwich, and O. Gutjahr, 2017: Extreme warming in the Kara Sea and Barents Sea during the winter period 2000-16. J. Clim., 30, 8913-8927, https://doi.org/10.1175/JCLI-D-16-0693.1
Kolstad, E.W., 2017: Higher ocean wind speeds during marine cold air outbreaks. Quarterly J. Roy. Meteor. Soc., 143, 2084-2092, https://doi.org/10.1002/qj.3068
Rampal, P., S. Bouillon, E. Ólason, and M. Morlighem, 2016: neXtSIM: a new Lagrangian sea ice model. The Cryosphere, 10, 1055-1073, https://doi.org/10.5194/tc-10-1055-2016
Wang, F., W. Li, and S. Wang, 2016: Polar cyclone identification from 4D climate data in a knowledge-driven visualization system. Climate, 4, https://doi.org/10.3390/cli4030043